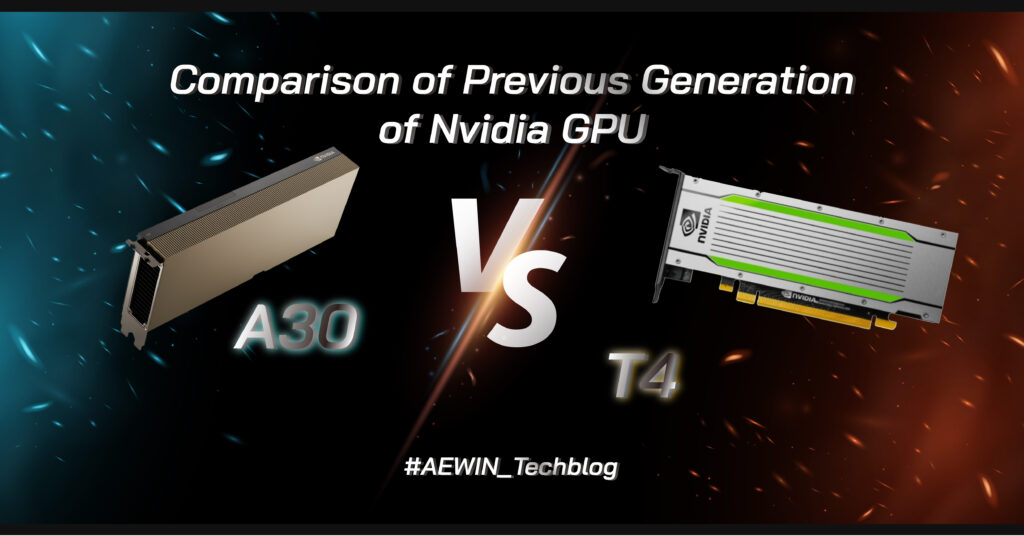
Nvidia just released their latest GPU with new architecture of Hopper and Ada Lovelace this Fall. Before we are getting deeper to know further details of the latest technologies, let us revisit the footprint of Nvidia previous microarchitectures.
Back in 2018, NVIDIA released an energy efficient T4 with revolutionary multi-precision performance which can offer extraordinary performance for edge inference. Three years later, A30 is in the market as one of the most versatile mainstream compute GPU for AI Inference. Let us figure out what is the difference between them.
Here is a brief comparison table with some specs without the performance results.
|
A30 |
T4 |
Process Technology |
7/8nm |
14nm |
PCIe |
Gen4 x16 |
Gen3 x16 |
TDP (Max thermal design power) |
165W |
70W |
Size (mm) |
268 x 111
(FHFL) |
170 x 69
(HHHL/low profile) |
Socket Width |
Double |
Single |
With the improvements of the process technology and PCIe speed, the performance of the A30 is expected to have a great leap forward which is align with the actual results. With bigger TDP and the larger size, the platform of supporting the GPUs shall be evolved along with it and up to date AEWIN platforms supporting both A30 and T4 are listed in the end of this tech blog for your reference.
In addition to the technology growth mentioned, NVIDIA has some innovations in A30 including the Architecture Tensor Core Technology.
Architecture Tensor Core Technology: Ampere vs Turing
Extra Enhancement |
Ampere |
Tuning |
Multi-Precision Computing |
FP64, TF32, FP32, BF16, FP16,
INT8, INT4 |
FP32, FP16,
INT8, INT4 |
T4 is powered by NVIDIA Turing Tensor Cores delivering revolutionary multi-precision performance (FP32, FP16, INT8, and INT4) to accelerate a wide range of modern applications, including machine learning, deep learning, and virtual desktops. In addition to the previous precisions, A30 is powered by NVIDIA Ampere Tensor Cores technology, supporting innovations including Tensor Float 32 (TF32), BFloat16 (BF16), and higher performance of the double-precision FP64. In addition to TF32 and BF16, A30 is with the new Multi-Instance GPU (MIG), let us take a closer look at them.
Tensor Float 32
TF32 is the math mode for handling the matrix math for AI/HPC applications. As shown in the following illustration, TF32 uses the same 10-bit mantissa as the FP16 math and adopts the same 8-bit exponent as FP32 to support the larger numeric range and more than sufficient margin for the precision requirements of AI workloads. Regarding TF32 deep learning performance, A30 deliver up to 10X higher performance over the NVIDIA T4 with zero code changes.
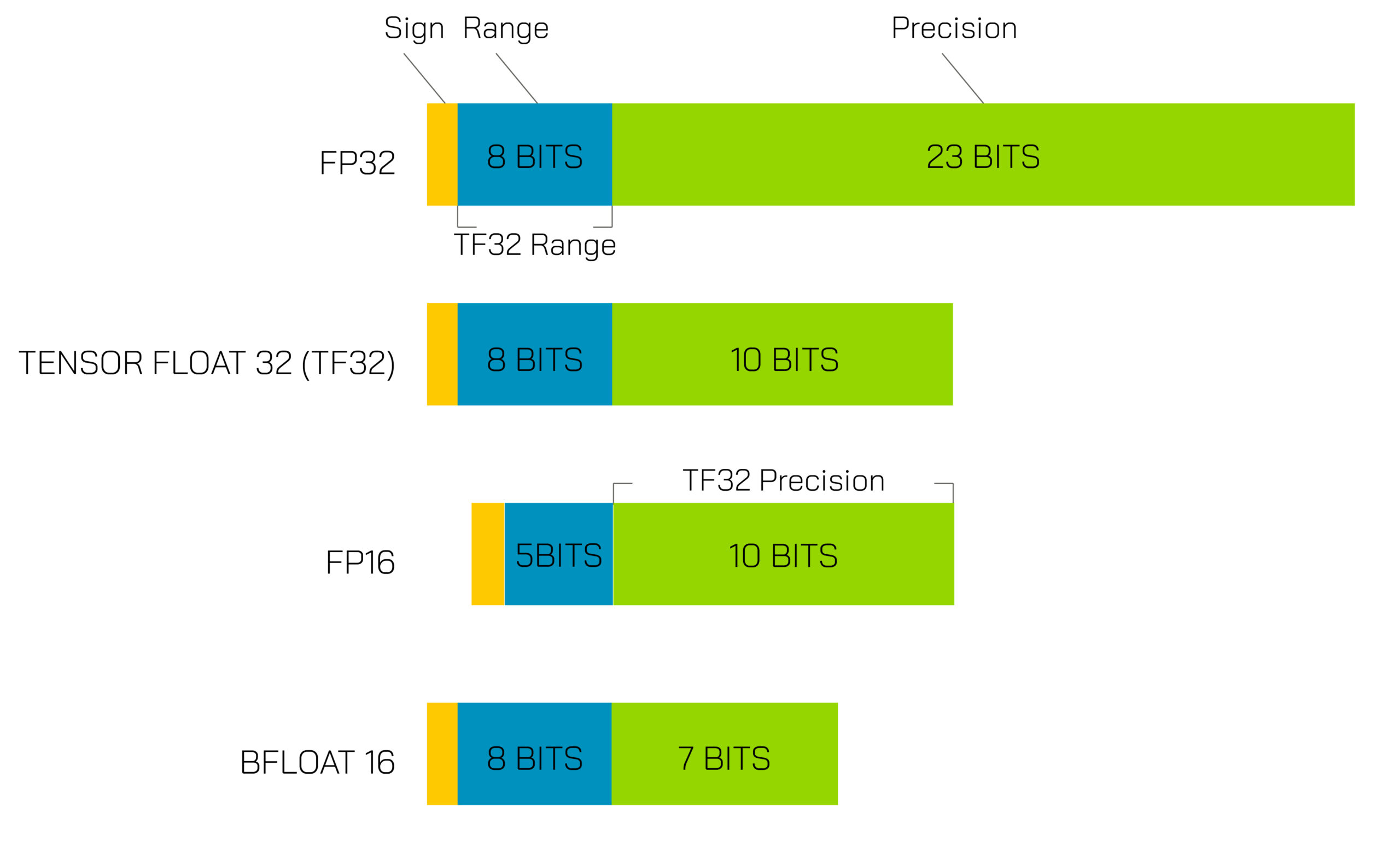
BFloat16
As for BF16, like we mentioned in our previous Tech Blog that it is essentially a FP32 with truncated significand bringing the performance of FP16 with the dynamic range of FP32 while using half the memory. With the reduced memory bandwidth, faster execution is permitted.
Multi-Instance GPU
The new feature of Multi-Instance GPU (MIG) allows GPUs based on the NVIDIA Ampere architecture to provide 933GB/s memory bandwidth which is almost three times higher than T4 (320GB/s).
Performance Comparison
The spec comparison of the performance results is as below.
|
A30 |
T4 |
CUDA Cores |
3804 |
2560 |
Tensor Cores |
224 |
320 |
Double-Precision (FP64) TFLOPS |
5.2 |
0.25 |
Tensor Float 32 (TF32) TFLOPS |
82/165* |
N/A |
Single-Precision (FP32) TFLOPS |
10.3 |
8.1 |
Tensor Perf. (Bfloat16) TFLOPS |
165/330* |
N/A |
Half-Precision (FP16) TFLOPS |
165/330* |
65 |
Integer Operations (INT8) TOPS |
330/661* |
130 |
Integer Operations (INT4) TOPS |
661/1321* |
260 |
Memory Bandwidth |
933GB/s |
320GB/s |
* With sparsity
AEWIN has verified A30 and T4 on AEWIN platforms including SCB-1932C, SCB-1937C, and BIS-3101. They share similar results with NVidia benchmarks.
Target market: Mainstream Compute/Inference vs ML/DL/Inference
We have seen the comparison between A30 and T4. Considering from the architecture to the performance, they shall be categorized as two levels of graphic cards and the target markets are different. According to NVIDIA’s announcement of the Data Center GPUs, A30 is for mainstream enterprise workloads like AI inference, training, and high-performance computing (HPC) while T4 focuses on edge inference with the advantage of compact size and small power consumption.
As AEWIN platforms also range from edge platforms to general purpose computing systems, to high performance servers, customers can select the most suitable one with the GPUs required for each application. Two of the recommended AEWIN Edge AI models are SCB-1932C and SCB-1937C, they are 2U, 2P servers supporting 2x FHFL GPUs and 4x NIC. To discover more, please don’t hesitate to talk to our friendly sales!
SCB-1932C: 2U Edge Server with dual Intel® 3rd Gen Ice Lake-SP, 2x dual slot Gen 4 x16 FHFL GPU cards, 4x PCIe Gen4 x8 slots for NICs, Accelerators & NVMe SSDs
SCB-1937C: 2U Edge Server with dual AMD EPYCTM 7000 series with 2x dual slot Gen 4 x16 FHFL GPU cards, 4x PCIe Gen4 x8 slots for NICs, Accelerators & NVMe SSDs
BIS-3101: Desktop Workstation with Intel 8th/9th Core i and 1x dual slot Gen 3 x 16 FHFL GPU card